
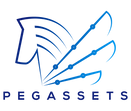

PEGASSETS
ENSEMBLE ACTIVE MANAGEMENT
=
Ensemble Methods
(predictive analytics technology)
+
Active Investment Management
THE NEXT EVOLUTION IN INVESTMENT MANAGEMENT
Get the best ideas from top managers all in a single portfolio. Ensemble Active Management (EAM) uses Ensemble Methods, the proven best practices of predictive analytics, to generate additional alpha unavailable from traditional active management. Research published by the CFA Institute quantifies the increased alpha at 400+ basis points annually.
The Problem
Active managers have failed to achieve their mandate to outperform their benchmarks on an after-fee basis, and the shortfall is structural.
How bad is the problem? According to the SPIVA (S&P Indices Versus Active) scorecard, 95% of all domestic active stock fund managers underperformed their respective benchmarks over the 20-year period ending December 31, 2021.
The Irony is that active managers’ highest conviction stock picks (overweights relative to the benchmark weight) do generate alpha, but unfortunately, most is lost before reaching clients.
The Solution = Ensemble Active Management
EAM builds on the past while embracing the future:
-
Grounded in core investment fundamentals.
-
Based on best practices for predictive analytics.
-
Uses Machine Learning technology to unlock the true potential of active management.
Ensemble Methods is the key lever to generating significant alpha within EAM Portfolios
Ensemble Methods succeeds by looking across multiple single-engine forecasts and mathematically identifying areas of agreement. These areas of agreement are then used to build a ‘super predictive engine’ with improved predictive outcomes. Ensemble Methods and the resulting superior multi-expert predictive engines is used by virtually every other industry in the world.
Two examples of the use of Ensemble Methods

-
2006 competition to improve Netflix's proprietary Cinematch.
-
$1 million prize for 1st team to achieve a 10% improvement.
-
40,000 entrants
-
Winner after 3 years used Ensemble Methods and 100+ underlying predictive engines.

Translating Best Practices of Predictive Analytics to Investment Management
-
Active managers' task is to predict or forecast which stocks will outperform.
-
To drive stock selection, managers build a decision-framework (i.e. a predictive engine). This encompasses their philosophy, process and views, or collectively, their bias.
-
In order to potentially outperform a benchmark, a manager must deviate from the benchmark (it's required). This creates cyclicality.
-
Periodic underperformance is a cost of bias; however, reducing bias creates higher variance, due to a mathematically-proven phenomenon known as the Bias-Variance Conflict.
By using several independent predictive engines (each with their own biases) and looking for agreement among them, you can dilute the impact of the bias without increasing the variance. This leads to improved accuracy. And in the case of stock selection, alpha.
To learn more about Ensemble Methods, see the white paper "Ensemble Active Management: The Next Evolution in Active Investment Management".
Executive Summary of white paper